Demystifying artificial intelligence: A brief overview
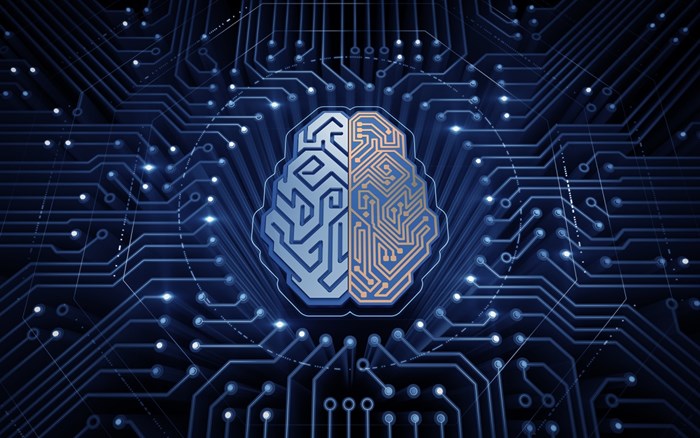
The integration of the best research into practice or evidence-based medicine is guiding daily clinical decision-making and greatly shaping surgical training. This evidence is built on data gathering, analysis and interpretation that can be substantially enhanced by the use of machines. A new and seductive research keyword has become artificial intelligence – and for many of us, this term resides in an unchartered territory of research and carries infinite yet poorly defined opportunities. What follows is the clarification of the meaning of AI and the illustration of its applications – as well as its limitations.
Artificial intelligence (AI) represents the study of algorithms from which machines can execute cognitive functions (e.g., problem solving, word recognition and more). AI encompasses machine learning, natural language processing, artificial neural networks and computer vision.
Machine learning (ML) provides predictions based on pattern recognition by combining mathematics and computer science. It can further be broken down into supervised and unsupervised learning. Supervised learning can be used to train an algorithm to predict a known result or outcome via partially labelled data. On the other hand, unsupervised learning can be useful to find patterns within the data. ML can aid in the identification, within large datasets, of small changes that may be undetectable for humans and not captured by conventional statistical-modeling strategies. ML algorithms can also be combined to reach previously inconceivable levels of accuracy.
Natural language processing (NLP), or the processing of the human language, allows machines "to infer meaning and sentiment from unstructured data." NLP has great potential for electronic medical records and physicians' clinical documentation, as it consolidates semantics and syntax by understanding and processing human-level language.
Artificial neural networks (ANN) simulate the nervous system by processing simple computational units (representing neurons). Deep learning networks are ANN with several layers. ANN have demonstrated that they can surpass the performance of traditional risk-prediction clinical calculators.
Computer vision is the machine understanding of images and videos, which can be applied to object recognition, image acquisition, image interpretation, image-guided surgery and more.
Although succinct of the various subfields of AI, this overview highlights its tremendous potential for clinical applications. Examples of AI applied to perioperative care and to plastic surgery are multiplying. Such reports of AI within the literature include the prediction of genetic risk for disease (e.g., non-syndromic orofacial cleft); preoperative surgical morbidity; healing time (e.g., using reflectance in burns); postoperative complications using electronic medical records; and surgical site infections. It has also been employed to assess, for example, the severity in cleft lip nasal deformities, craniosynostosis head shapes and tissue perfusion in microsurgery.
Promising – though cautions remain
Although very promising, it remains crucial to recognize the limitations of AI and avoid unrealistic expectations. Furthermore, some skepticism already exists regarding entrusting machines to perform cognitive functions that would apply to healthcare delivery – and that physicians could achieve with possibly more accuracy. Integration into clinical practice may initially be limited by liability as well as financial requirements (reimbursement of a machine model and implications for physicians).
AI may also bring some disruption to the healthcare workforce in its current form, and physician adaptation to learning new skills will likely be required. Physician-machine interactions would appear essential in many circumstances to maintain accuracy, diminish costs and maximize output. For example, although ML can develop robust risk models, liability remains a barrier to implementation. By enabling machine and physician interactions, the machine can have greater sensitivity (to avoid missing true positives) and physicians can contribute to higher specificity (identifying true negatives), for an efficient and optimal model of care.
The premise of having access to AI algorithms implies having access to large amounts of unbiased data in order to achieve acceptable performance levels. Collection of such volume of data in a quality fashion is imperative to promote generalizability of findings – which may represent a challenge and introduce additional burdens to the system. Similarly, particular attention to the appropriateness of the clinical inquiry as it relates to the available data will be necessary.
The emergence of AI in daily healthcare delivery is taking place and will likely be omnipresent in the future. Translation of large amounts of data into knowledge has the potential to transform diagnostic accuracy, prognostication, imaging analysis and more. Opportunities to explore AI within plastic surgery for the provision of cutting-edge care are promising – and this should continue to be encouraged.
Dr. Roy is a plastic and reconstructive surgery resident at the University of Toronto with a research interest in AI and its future clinical applications.